Earth System Models (ESM) simulations represent one of the most challenging HPC use cases due to very high computational cost, intensive Input/Output patterns, very large data volumes produced, and the necessity of post-processing them to distill knowledge and extract relevant information. Novel workflow solutions are therefore required.
The atmospheric, ocean, and later Earth System Models (ESM) simulations on High Performance Computing (HPC) architectures (e.g., supercomputers) have a long history, dating back to the 1960s. This is one of the most challenging HPC use cases, not only for the very high computational cost, but also due to additional challenges related to intensive Input/Output patterns, the production of very large data volumes, and the necessity of not only producing data on HPC, but also post-processing it there in order to distill knowledge and extract relevant information.
The overarching goal of Pillar II is to enhance innovation for intelligent and integrated Earth System Model workflows exploiting novel techniques of Data Analytics on HPC infrastructures (HPDA) and AI (i.e., Machine Learning based) approaches.
Figure 1. Overall Pillar II workflow
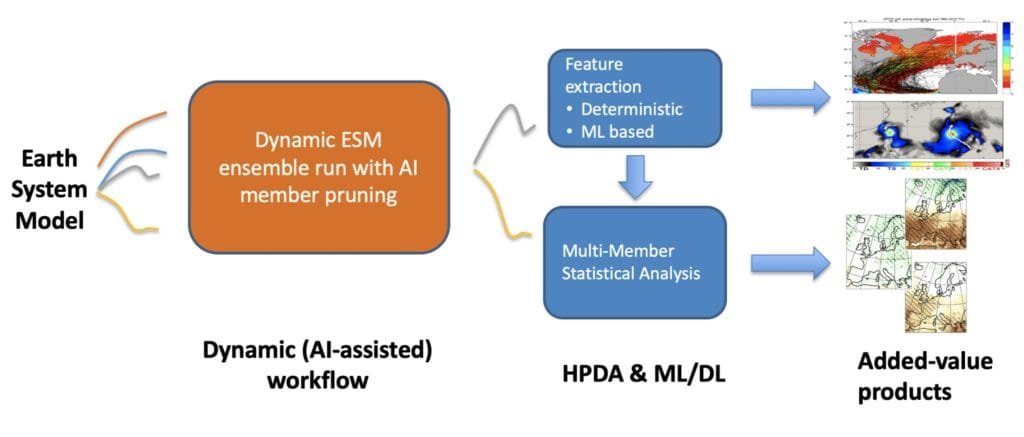
A typical ESM ensemble workflow experiment consists of several start dates and members divided into several sequential simulation chunks. A strong and performing workflow solution is required because these types of experiments are very resource consuming and, due to their static nature, it is very hard to have a flexible approach.
The overall idea is that eFlows4HPC provides the components or functionalities to allow ESM simulation runs the capacity to prune members that do not add anything useful to the whole simulation. The idea is to make a better use of computational and storage resources by performing the smart (AI-driven) pruning of ensemble members at runtime and releasing computational resources accordingly, exploiting novel and high performance software solutions to access data stores for big data applications (e.g., Hecuba).
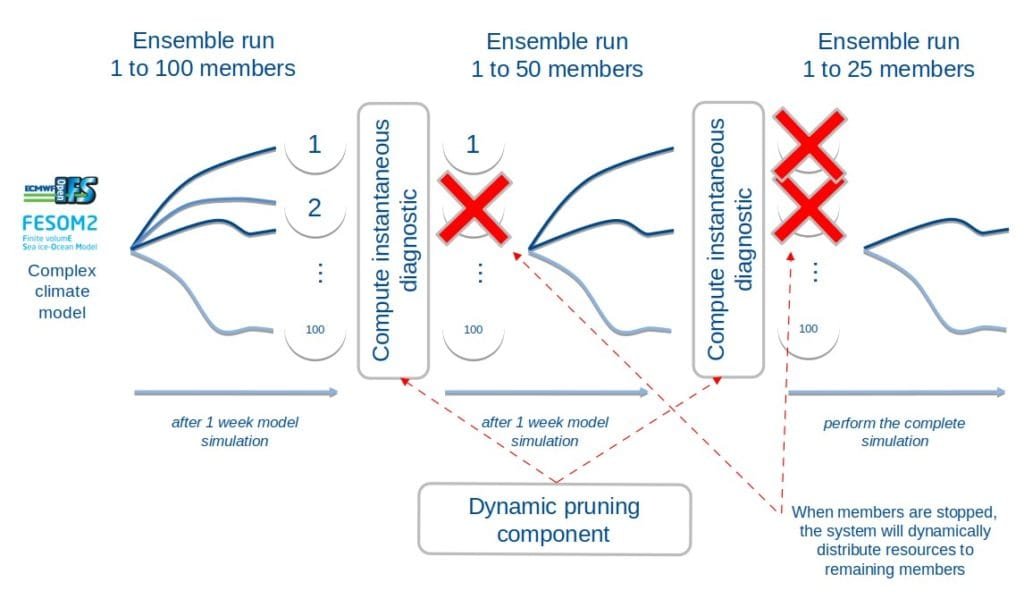
Figure 2: Dynamic ESM AI-Assisted workflow
On the other hand, data-driven solutions provide new methodologies for analytics and feature extraction at scale, for example with respect to multi-model analysis (i.e., simultaneous analysis of datasets coming from different climate models) and extreme events (e.g., Tropical Cyclones) analysis.
In order to evaluate how TCs activity might change under different climate conditions – in terms of landfall, associated strong winds, heavy precipitation etc. [1] – it is important to investigate their representation by General Circulation Models (GCMs). Also, our knowledge of the TC interaction with the climate system can build on GCM results [2]. This will be done by following different TC detection and tracking methods available in literature and also by investigating new Machine Learning approaches, to verify the possibility of speeding up the detection process in the context of a multi-model multi-member analysis.
The eFlows4HPC infrastructure will be exploited in the context of the case study related to the multi-model analysis of a Tropical Cyclones (TC) track also leveraging on scientific frameworks for data analytics in HPC context (e.g., Ophidia).
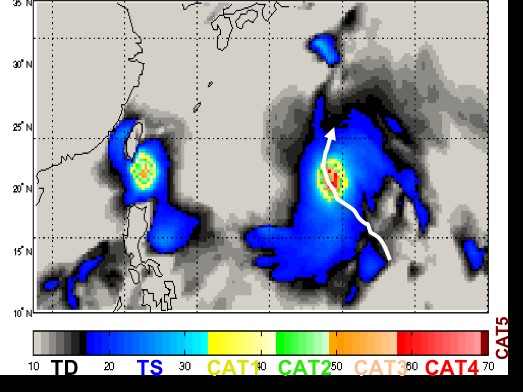
Figure 3. Example of a West North Pacific Tropical Cyclone Track and the associated winds in a ¼ degree resolution General Circulation Model (CMCC-CM2-VHR4). Color shading indicates wind velocity [m/s] and the associated class from the Saffir-Simpson scale
Publications:
[1] Villarini G., D.A. Lavers, E. Scoccimarro, M. Zhao, M.F. Wehner, G. Vecchi, T. Knutson, 2014: Sensitivity of Tropical Cyclone Rainfall to Idealized Global Scale Forcings Journal of Climate, doi: 10.1175/JCLI-D-13-00780.1
[2] Scoccimarro E., S. Gualdi, A. Navarra, 2012: Tropical Cyclone Effects on Arctic Sea Ice Variability. Geophysical Research Letters, 39, L17704, doi:10.1029/2012GL052987
[3] D. Elia, S. Fiore and G. Aloisio, “Towards HPC and Big Data Analytics Convergence: Design and Experimental Evaluation of a HPDA Framework for eScience at Scale,” in IEEE Access, vol. 9, pp. 73307-73326, 2021, doi: 10.1109/ACCESS.2021.3079139.